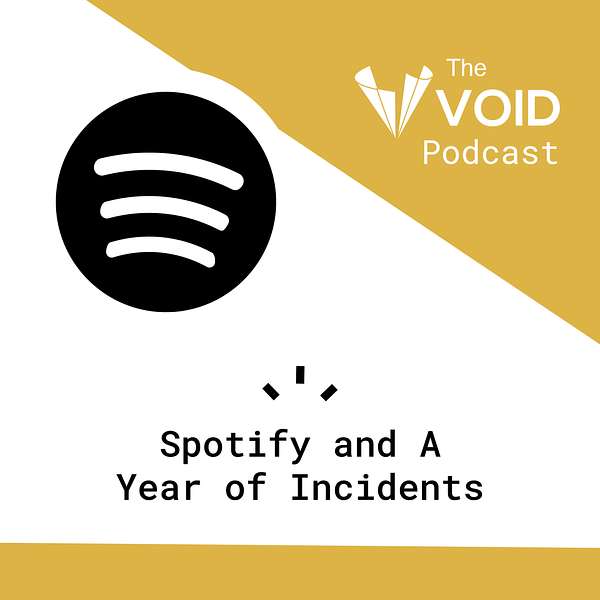
The VOID
The VOID makes public software-related incident reports available to everyone, raising awareness and increasing understanding of software-based failures in order to make the internet a more resilient and safe place. This podcast is an insider's look at software-related incident reports. Each episode, we pull an incident report from the VOID (https://www.thevoid.community/), and invite the author(s) on to discuss their experience both with the incident itself, and the also the process of analyzing and writing it up for others to lean from.
The VOID
Episode 3: Spotify and A Year of Incidents
If you or anyone you know has listened to Spotify, you're likely familiar with their year end Wrapped tradition. You get a viral, shareable little summary of your favorite songs, albums and artists from the year. In this episode, I chat with Clint Byrum, an engineer whose team helps keep Spotify for Artists running, which in turn keeps well, Spotify running.
Each year, the team looks back at the incidents they've had in their own form of Wrapped. They tested hypotheses with incident data that they've collected, found some interesting results and patterns, and helped push their team and larger organization to better understand what they can learn from incidents and how they can make their systems better support artists on their platform.
We discussed:
- Metrics, both good and bad
- Moving away from MTTR after they found it to be unreliable
- How incident analysis is akin to archeology
- Getting managers/executives interested in incident reviews
- The value of studying near misses along with actual incidents
Welcome to the VOID podcast. I'm your host, Courtney Nash. If you or anyone you know really has listened to Spotify, you're likely familiar with their year end Wrapped tradition. You get a viral, shareable little summary of your favorite songs, albums and artists from the year. Or if you're like me, and sometimes you let your kids play DJ in the car on road trips, the algorithm can seem very off from your own personal taste in music. But I can confirm we're all huge fans of Lizzo, so we've got that in common at least. Today, we're chatting with Clint Byrum, an engineer whose team helps keep Spotify for Artists running, which in turn keeps well, Spotify running. No pressure. Right? Each year, the team looks back at the incidents they've had in their own form of Wrapped. They tested hypotheses with incident data that they've collected, found some interesting results and patterns, and helped push their team and larger organization to better understand what they can learn from incidents and how they can make their systems better support artists on their platform. Let's get into it. I am so excited to be joined on today's VOID podcast by Clint Byrum, Staff Engineer at Spotify. Thank you so much for joining me, Clint.
Clint Byrum:Oh, it's great to be here. Thanks for having me
Courtney Nash:So we are going to be chatting today, about a multi incident review that you published back in May earlier this year. I'll put the link to that in the notes for the podcast. Your team decided to look back at a bunch of incidents from 2021. And so I was hoping you could talk about what led the team to wanting to do a more comprehensive view of that set of, of incidents from last year
Clint Byrum:Yeah. My focus and my team's focus is on the reliability of Spotify for Artists. So most people are familiar with the consumer app that plays music and podcasts, and does those sorts of things for you. But Spotify for Artists is sort of that back office application that when you want to manage your presence on the application, or if you want to publish music or some some other things where you're, you're, you're more of a in work mode rather than listening to music mode. That's where you come to us. And so my team, which is I'm proud to say we got to call ourselves R2D2'cause we're the droids that do the reliability work. The company has a very rich platform that's centralized for the consumer application. And most of it works for the back office app too, but you can imagine we're like lower scale, but higher consequence. And so some of the, some of our practices and testing focuses are different, and my squad sort of helps everybody around— we call it the music mission— do those things in a reliable way and take care of their uptime and, and do better incident reviews and things like that.
Courtney Nash:So what, what led to that, that team then looking at incidents in order to try to have a better experience for the users of that particular platform?
Clint Byrum:Yeah, you, you might be familiar. Spotify has this tradition of"Wrapped" where if you're a user of Spotify, once a year, we send you a cool little packet of like, this is what you listened to last year. And this is you know, your favorite artist and you're most like, These people over here. It, it's kind of a tradition at the end of the year that Spotify looks back at the, at the previous year. And so we started that a couple years ago before I was actually even on the team. It was just a working group of some reliability focused engineers where we just stopped and looked back at all the incidents that people declared for a year and try to learn from them. We've gotten better. At the beginning, it was just sort of looking at them and making sure that we understood like how many there were and what were the, what was our mean time to recovery. If we could discern that you know, basic, basic discerning things. But the last few years we've been trying to do real science. So we've sort of made hypotheses and said, we think that this was true last year. Or we think that these two aspects played against each other and then we design. Little pieces of data that we're looking for in each incident. And we go looking for them and when we find them, we catalog it and see if we can learn something from, from the aggregate, rather than just looking at individual. incidents
Courtney Nash:So that my next question was specifically going to be about hypotheses. So you mentioned having some, can you say more about what some of the hypotheses your team generated were and, and how you came up with them?
Clint Byrum:Yeah. So the, in the public post you, you're seeing sort of the best ones, like the things where we think we can learn the most from, and they're relevant to maybe not everybody at Spotify. So we had a hypothesis, our team maintains this web oriented synthetic testing suite. So I call it it's sort of like droids making droids. We, we help the teams that are responsible for features write tests that drive a web browser and just go click around on the live site and make sure that still works. And we had a hypothesis that when you don't have a test for your feature, it would take much longer to detect an outage. That seems obvious, but, well, we sort of were wondering like, is this true? Is our value proposition actually real? I think that's a pretty easy, we thought it would be, you know, pretty easy to, to prove. And, and it was For instance, you you'll see in the, in the post. We found that essentially when you have a test and you, and, and then the feature is able to be tested in this way. It's about 10 times faster that you find out because you're not waiting for a person to notice or metrics. Sometimes metrics don't tell the story until it's really, really broken. And when you, don't, you're waiting for all those other things to, to dominoes, to fall. And actually quite often it's just support that's gonna find out because it's not like a metric or a scale problem for us. A lot of times it's just features interacting poorly
Courtney Nash:I guess better support than on Twitter. But yeah, better, even more upstream from that. Right. Actually it's interesting, cuz Twitter, we do have metrics that are, you know, sort of like when people are talking about Spotify or Spotify for artists on Twitter. And so those are, you know, something that we use as an early signal, sometimes even the best monitoring fails, Yep.
Clint Byrum:It's interesting because we've gone on a journey that, you know, this was done in December of 2021. We published in may since then, we've even watched your talk at SREcon where you talked about meantime to recover and, and we've sort of let go of our dependence on metrics like that, but we were looking for, how can we get better fidelity? As we looked in the sample, various small sample of the data, we were finding wildly inaccurate and we actually proved that one out as well. People don't enter start and end times. So that's the kind of stuff we were looking for.
Courtney Nash:You can imagine I was very honed in, on, on the TTR stuff in the report. For anyone who hasn't listened to me rant about that before. I can, I can post you, I'll put some links to that in here and, and I'll link to the talk that, that Clint is referencing. One of the things that we've seen in the void obviously is that those kinds of duration data are all over the place. And so I'd noticed that you said that, I'm super glad that you all are, are moving away from Some incident nerd type folks like us often like to call"shallow metrics" hat tip John Allspaw for that term. But I've always harbored hypothesis, I guess we'll say since we're in hypothesis land, that time to detect data might actually be more useful and that's kind of what you're talking about here, right? I harp on meantime to you know, sort of remediate or whatever you wanna call it because it, it's not a normal distribution of data. And that's probably what you found, which is why yours were all over the place. But I wonder if the detection times are more bell curved. Right? I wonder if that slice of the, because it's really, and especially when you can have synthetic tests and those kinds of things that you, you are in that world where your data are normal distribution and you could find improvements in them and you could say, oh, a 10% difference in the average is meaningful. Versus the variability that you see in the actual aftermath of an incident happening. I thought that was really still kind of what was lurking in. in your data there. So I thought that was a really interesting piece of it.
Clint Byrum:I. I think, I think you're onto something. And I see that as well in the conversations that have gone on after this post and people sort of notice it and, and we'll pick it up and, and, and come and reach out. There's a lot of curiosity about that, and a lot of people pointing out that really what you did was show a normal distribution in, in time to detect, as you said. And also our data scientist was like just super excited to get into some of the weeds in the data and, and wanted us to do some more collection because he was able to show that we, we, we really had proved this. We looked at some of the other variables that we had reliably collected and we found that, no, this is, this is something that's really tugging it in that one direction in, in a really hard way, and I think you're right. One of the things that I got from not just what you're saying, but John Allspaw and, our learning from incidents community is that these aggregates they're, they're easy to read, but they're not so easy to discern real value from. So it's, it's often easy to like look at the, the dashboard and say, oh, meantime to recovery, it's in good shape, or it's not so great. Need to, you know, get the hammer out.
Courtney Nash:Pay no attention to the man behind the curtain, right?
Clint Byrum:Right. But when you start to break them down into time to detect and time to, to, to respond and, and sort of also like, there's this idea of like, when is it over? That's another really interesting question that we got into while we were doing this study, like, what are we going to decide is an incident is over, are we recovered? Because oftentimes you're mitigated, but you still have a degraded system that is either costing a lot to run or may be at a high risk to, you know fall over again. So those, all those questions, like having smaller metrics that end timelines to look at actually allows us to learn more. Whereas the aggregate wasn't really telling us anything. And I, I wanna add one more thing. I think, I don't remember who said this. Somebody said it in the Slack— I think you might be there on there too. Looking at meantime to recovery and trying to learn anything just from that is like looking at the presents under the Christmas tree and trying to guess what's in them
Courtney Nash:Yeah.
Clint Byrum:like there's
Courtney Nash:I used to, yeah. I used to put rocks in big boxes for my brother when at Christmas, cuz I was a jerk.
Clint Byrum:Right. Right. Or even like in, in the Christmas story, right? The BB gun is hidden behind the piano to throw him off. And there's a little bit of that where like, like we, we opened the presents and we looked in and actually saw what was there. We saw a very different story than what we were guessing we would see.
Courtney Nash:There was one other little note in that section. You know, you mentioned people weren't recording these things and, you said something like this isn't a failing of operators, but a failing of the system." Can you talk a little bit more about what you meant by that?
Clint Byrum:Yeah, I'm a, I'm a big believer in that, axiom, that, that people don't fail, the system and cognitive work, the system fails them. And in this particular case, we were asking people when they were, so we used JIRA for cataloging incidents and, and managing them—which is a whole can of worms that I rather not get into— but it does have a form that we ask people to fill out when they're done, when they're sort of marking it as moving into the post incident phase. And it has a start and end time it's it doesn't ask you many questions. It sort of like asks you to make a, some, some, you know, tags and, and some short description. And then when did it start and when did it end? And it has defaults and those defaults are very popular. So
Courtney Nash:As, as so many defaults are right?
Clint Byrum:Right, right. Because that's one less thing to go go out and, and, and dig through. We have a correcting practice for it, which is that we do ask people in that post incident phase to build a timeline so that we can have a post incident review and talk about what actually happened. And those are often very accurate. So when we went and actually went and read through the timelines, you could go through and find the start and the end. So when we corrected those, we kind of that's one of the things we were looking at is how far did we have to correct them? And in fact, that was meaningless. What we found was they're just always those default values that kind of come from when you opened it, or when you touched the, the incident in a certain way.
Courtney Nash:Yeah. And I mean that you can place yourself in the mindset of a human who is just finished managing an incident and yeah, probably don't wanna have to do that much anymore at that point.
Clint Byrum:Yeah, I called it"paperwork" in the post and I stand by that. Not just start an end time, but even just getting to that form, which is where we move the, to the post incident phase. People often take days to get back to that because it is a stressful event whether you were able to mitigate and go back to sleep and maybe handle it the, the next business day or however you got to that point where oftentimes people just completely move on. It's a, you know, it didn't have a big impact. Maybe there's not a lot of people with questions and we actually have a nag bot that comes and tells people if they haven't closed an incident for a while, it'll come back and poke them. And that's usually when they're filling out that form.
Courtney Nash:That's so funny. You know, we, we don't see a lot of these kinds of multi incident reviews out in the world. I, I feel like honeycomb is a company I've seen some from and, and a few other places have done it. So there's not a lot of precedent for how one does such things. And I'm sort of curious what your methodology was for how you approached that.
Clint Byrum:Yeah, I, I agree. I it was something that surprised me when I arrived. So I, it had been done two years prior when I, when I got to Spotify and that torch had been handed from this reliability working group to this full-time squad. And so we thought it, we liked what we saw. And so we, we decided to repeat it, but it was interesting because most of the people from the working group, it sort of moved on and weren't available to us. So we had to look at the results and work backwards to the methodology. So we invented some of it, our, our, ourselves. Our main centering principle was that we are gonna time box the amount of time that we spend with each incident and that we're not going to change them in anyway. So we may make notes that we couldn't find something or we don't have much confidence. That's actually a score that we added, but we're just gonna go back and look. We really wanted to change them by the way we could see glaring errors in the paperwork, but we just said, that's not what we're doing. We're just looking at them. So we set a time box. We looked at the number of incidents, decided the two of us who were doing the analysis at the time, we have 16 hours total for this, divide the number of incidents, add a little slack time and say, okay, once you've reached that, if you haven't gotten enough confidence in what you're looking for, then you mark it as a low confidence incident and we move on. So we just dumped it all in a spreadsheet and assigned them to one analyst or another. Sometimes we did them in pairs and we started just moving through what we thought would be interesting things to look at. So it changes with each study. We were looking at things like how how complex did this seem or how many people got involved with it? The first time we did it, we tried to do time to recovery and we found that we were spending too much time reading timelines. Also whether or not there was a post incident review that was actually just like on a whim. We just thought, well, I see a few that it hadn't happened. We didn't realize just how many incidents ended up not getting that time spent. And that was something that really troubled us the first year. So we did a lot of work the next year as a squad to advocate for those reviews. And then the rate did go up. It's still not where we want it to be. I'd like it to be a hundred percent. But that was the idea,was go through each one, look at you know, these aspects that you're trying to find based on the hypotheses. I forgot to mention that first we make hypotheses. Then we pick metrics, then we look for them. And at the end we'd spend a few times having a third person come and spot check just to make sure we were applying it. And then from there, we did some data analysis, worked with our data scientists to make sure we're not inventing statistics, because none of us are statisticians. And we totally did that... at first. But luckily we have data scientists around who are very happy to like get into it and, and were like,"Yeah, you can't really say that." And then produce a report with some, with some findings.
Courtney Nash:What were you looking at? What materials were you looking at? Just the post incident reviews. Were you, I mean... Grafana? I don't know what y'all use... outputs. Like what gave you the, the sort of data for those hypotheses that other than... I at a lot of timelines.
Clint Byrum:That's a great question. So there's a single JIRA project for all incidents at Spotify. And then we narrow that down to the ones that have been tagged as related to our product, which is actually pretty reliable, just because it's one of the ways we communicate sort of, if you declare an incident against Spotify for artists, then some stakeholders get notified. So it's pretty important that it happens. So we, we first just pulled out the raw data from JIRA and, and that has a description. Some actually teams will actually manage the incident directly in JIRA. So they'll, they'll enter comments through the way. What's really common though, is they'll just drop a, a Slack link in there. And so in the time box we had, I think like this last year, we had 30 minutes to look at each incident, which is actually pretty generous. Whatever we can find related to the incident in 30 minutes. So we start at the JIRA ticket, but you'll find there's a post incident review document sometimes they're recorded, so we'll try to watch a few minutes of it. You'll find Slack conversations. If there's really not a lot, we would do a little bit of searching, but in you'd usually hit the time box pretty quickly, if that was the case. And then we had like a one to five scale in confidence. And so we pretty much anything you see mentioned in that study, we threw out everything under four. So like fours and fives were like, we're really confident about that we captured the data. There's actually not a lot of ones and twos and there's just a few threes. Most people put enough in there that we could make some calls about them.
Courtney Nash:This feeling I've had about people who do your kind of work and, and other people who are getting hired even as incident analysts. You're like you you're like complex system archeologists. I mean, you're just going back and trying to look at tunnels and scratchings on the wall and, and sort of figure out really what happened. When you would go in, like you'd mentioned that there would be like a Slack link. Were you lucky enough that there would be like an incident channel or does a channel get declared for incidents and like everything's in one place? Or what did, what did that look like for you all.
Clint Byrum:Would that they were always true. Yes, sometimes there there's a specific channel created for an incident. Generally, the more severe or broader impact incidents get those channels created. It wasn't always the case. I would say actually in 2021 is when we started doing it more as a matter of course, but there's no sort of manual that says,"Make a channel and number it this way," it's sort of a tribal knowledge that's works out well for everyone. A lot of times, teams that maintain systems or on-call rotations that share systems—sometimes that happens— will have a single channel where they're like, sort of having all of the slack alerts from PagerDuty or from Grafana or whatever system they're using to alert them will, will sort of coalesce there. And then they open threads against them and discuss. So sometimes you just get a thread and sometimes you get a whole channel. It just depends on how sort of broad or narrow it was and how well managed the incident was. At times you get very, very little, but the incident review is so good that you're able to just read that document. One of the magical and frustrating things about Spotify is that, well, we have a lot of autonomy, how you do your job is your choice as, as a squad. So a small group of 10 people make that choice. And as a result when you try to look at things across any broad swath of the company, even just across those that work on Spotify for Artists, expecting things to be uniform is probably a mistake.
Courtney Nash:Well, I think expecting things to be uniform in general is probably a mistake?
Clint Byrum:Probably yes.
Courtney Nash:In any system, honestly? But yeah, I mean, that sounds like that would be, you know, a bit more challenging. And so you didn't interview people yourselves, then that may have happened in the post incident reviews, but not in the work you all did.
Clint Byrum:Yeah, not as part of this, although, because we do some of that at the company, we try to go deeper on certain incidents. Those are the fives on that confidence score, where we have a really well written narrative report where maybe a few analysts have gone in and, and gone over things with the fine tooth comb and had a really effective post incident review. So I think we had out of the a hundred or so that we looked at, I think we had five or six of those and those are so easy to read that you can get that done in five minutes. I wish we had the time to do that on every incident, but it's, it's, it's a heavyweight process, but it makes it for a really fantastic result for the archeologists.
Courtney Nash:So you had your hypotheses, but while you were doing this, did it reveal any other sort of patterns or repeating themes that you weren't expecting... or?
Clint Byrum:I wish that we had a little more time to do these and to look for exactly that. While we're going through them, we sort of by osmosis noticed some stuff, and that is another sort of unstated reason to do this. Sure, it was great to have statistics and, and to be able to make some really confident calls about how incidents are managed at Spotify, but from a perspective of a squad that's trying to help people be better at being reliable and resilient and they're building their systems. We certainly got a good broad picture of what's going well and what's not. I think if we actually sat out and didn't do the statistical approach and just tried to find themes in them with the same amount of time, we probably would have an equally interesting, although very different report to, to write.
Courtney Nash:Interesting. How is the report received internally? I'm curious some, you know, some places it's like, well, people read it and they said it was great.
Clint Byrum:Yeah. It's it's been interesting. Our, our, our leadership really loves this thing, so they have become, have come to expect it. In the in the winter they, you know, they, they're sort of like, oh, are you working on a study this year? Like, you know, what are you, what are you taking a look at? Like, did you, did you ask this question this time? So they usually kind of remember when they get back from a winter break that we'll be, probably be publishing it. And that's very gratifying that, they're paying attention. I think also it just tells that we're giving them sort of things to keep an eye on from a reliability perspective. Engineers also tend to see it as a sort of an interesting, curious moment to, to, to reflect on where they sit on it. So I've, I've talked to a few engineers who are you know, had a few incidents through the year and, and, and they're curious to see, like, they, they go look at the spreadsheet and they, they wanna see how their incident was rated.
Courtney Nash:Oh,
Clint Byrum:like, yeah. So they're, they're, they're, they're digging around in the ones that they remember and, and, and they're sort of seeing like, oh, that, yeah, I don't know. Is that really complex? Did we get a lot of what, you know, that sort of engineering. A lot of also what happens is people will go back and fill in a lot of details because we'll mark their incident as low confidence where like, we couldn't find an incident review document, or we didn't see the slack channel. We don't go back and revise the report, but it's always interesting to get a ping, like,"Hey, I saw that, you know, this was a really big incident and I, I forgot to put the link here. So here it is in case you're curious." So we get, we get all, all kinds of engagement from it. I think there are a few hundred engineers who work on Spotify for artists and I I think we, we saw that about 60 of them read the report. So I think that's a pretty good rate of of at least opening.
Courtney Nash:yeah. Well, by marketing standards, that's a smashing open rate. But that, engineers are reading that maybe a little bit out of terror, I suppose, but hopefully not. It seems like they see it largely in a, in a positive way. It's like, yeah, you guys need a yearbook: the Spotify 2021 incident yearbook
Clint Byrum:I like that. Yeah.
Courtney Nash:"Most likely to never occur again" or so we think...
Clint Byrum:Oh, I'm totally stealing that. It's it's gonna be in this one. Like I said, we wanna make it work like Wrapped. So Wrapped is this very visceral visual experience, audio experience where, you know, we kind of own that dance, social media for, for music. And we're always like, oh, we're gonna make an Incident Wrapped. And usually it's just three cheeky slides in some sort of all hands that drive people to the report. But that seems to get the job done.
Courtney Nash:So it was just the, it was just two of you then doing the meta-analysis. And were either of you involved in any of those incidents, or no? Did you go back and see things that you had been a part of?
Clint Byrum:Yeah, for sure. We get involved in incidents. We do maintain that testing framework and that's often broken itself. You know, there are false positives. We get involved when that's happening. Or in, in some cases like one interesting aspect is that so we have a web test that will just sort of log in and sort of add somebody to a team and remove them to make sure that that works. Well, that is an auditable event. We wanna know when that happens because it has security ramifications. And so our test suite filled up the audit log database. So we got involved with that one, for instance, that was a lot of fun. And so we had to, and, but what actually was really fun about that is the result wasn't that, you know, sort of, we had to stop doing it so often, the team that was maintaining it realized that actually the purging process that had been designed in just wasn't working and no other user on the system had ever done as many things as the synthetic test users. So they, they were able to actually fix something. I love incidents like that— how deep can we go in finding things that aren't what we think they are?
Courtney Nash:Yeah work as imagined versus work as done or systems as we think they work and how they actually work. What was it like to go back and look at some of those? Did the memory fade was the, you know, was, was looking back at it at all interesting for you just to personally, from having been involved in some of those?
Clint Byrum:I, I love looking back. Time, you know, really does sort of set the memories into your. Sort of neural network of concepts, right? So there, there's some sort of proof that people remember things best when they've had a little time to forget them and then are reminded of them. And so I certainly do remember like that one, for instance It happened. It was over fairly quickly. And, and honestly wasn't all that complex, but then going back and reading the post incident review, I remembered the conversation and I talked to one of the other engineers again, and they were like,"Oh yeah, we, we did do that work. And here, look, this is how, you know, we found this too." And so going back and revisiting them definitely was, was a, was a good experience for me personally. And I know from other engineers who've said similarly to the leaders, like when is that coming out? But they've had same thing. They're, they're usually looking for a specific one that, you know, maybe had an emotional impact for them or that they just are curious about. But yeah, it's a chance to look back and I think that's really valuable.
Courtney Nash:It all is so much time to do, but you mentioned that at the very beginning of, of the post, that we trade productivity now for better productivity later. Right. And I think a lot of organizations struggle with justifying that kind of work. You know, so it's not always easy to draw the direct line from that, from that fuzzy front end of, of it analyzing these things to like really clear outcomes, every time.
Clint Byrum:Yeah. I say that out loud quite a bit, actually. Whenever we're discussing incidents in general that this is intended that these failures are things that we want you to have as an engineer. We have an error budget, and that doesn't mean that it's, oh, if something goes wrong, you can spend up to this amount. We actually want people to push up to the edge and spend that error budget. If they're not, that's also a signal they're not taking enough risks or that they're spending too much time on the system health, which will blow some people's minds to say it that way. But it's, it's absolutely, I mean, it very much, when I say that we are spending that incident time to learn about the system in ways that would just not really be possible if we simulated it, or if we try to think through and, and planned for everything, it would be too costly. It is okay to have a few minutes where the users are inconvenienced. We're sorry, users. Because we are building so much more value when we're actually spending the time after that to learn. How's it gonna break again? Where is it weak? Maybe it needs even more work or less of doing well, so really important.
Courtney Nash:What are your plans for this year for your post? Are you thinking about it yet? Are you gonna do anything differently? What are you looking out towards for Spotify Incident Wrapped 2022?
Clint Byrum:Yeah. At this point, it's become a tradition. So I think we will definitely look back at the year of incidents again. We had a plan to sort of do more work before we get to the end of the year so that we could maybe spend the time box going a little more in depth. So spend a little bit all through the year, rather than all of it at the end that has proven difficult to, to find time to do. We also thought about maybe crowdsourcing some of this and asking people to fill in some more forms. We realized they already aren't doing the paperwork we're asking them to do yet. So until we come up with an incentive for that we've kind of put that on the shelf, although we still would like to do it. I think this year, we're gonna focus a little bit less on these metrics and looking at you know, trying to like find aggregate clues here. And we're really trying to find the incentives that drive people through the process to get that value of the post incident review and, and during, and the learnings of fixing things when they're really badly broken. Because we, we, one signal that we also got, that's maybe not in the post very much, is that there, there are a number of incidents that would be very useful and valuable to learn from that are never declared because they're near misses or they didn't rise to the level where they needed to be communicated about. And we really, really would like people to be able to declare those, or record those somewhere and just give us that quick learning. That's proven very difficult because our, our process is heavyweight. It needs to be but we, you know, we'd like to figure out a way to do that. So we're gonna look into, you know, how we, how we can maybe find those the, the magic time where it's still happening, even despite the heaviness of the process and, and, and do more of that.
Courtney Nash:That's very exciting to hear. I am a big fan of near misses, but I also fully understand how that is one of the hardest sources of information to incentivize and, and collect. So I wish you well, and I hope I get to hear about some of that in 2023.
Clint Byrum:Is that already almost coming? Oh my gosh.
Courtney Nash:I mean, if you're gonna write it up, it'll come out next year. So I'm not saying it's anywhere near news. No, so no pressure. Well, thank you so much for joining me today, Clint. It's been really a treat to have you on the podcast.
Clint Byrum:Yeah, it's been great. Thank you.